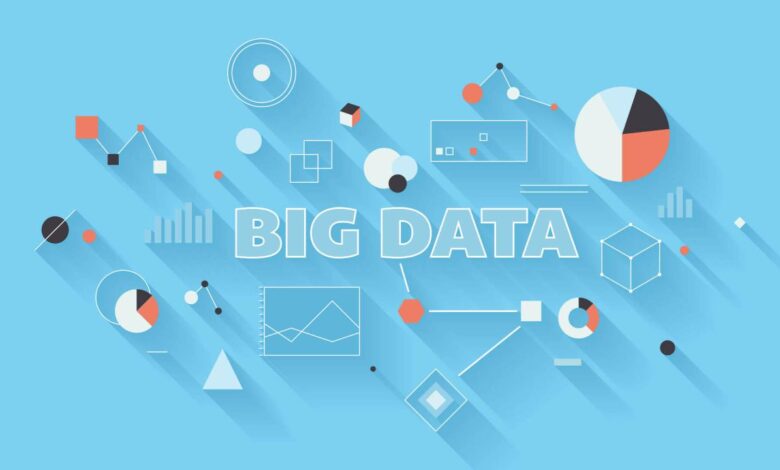
Big Data Little Data A Deep Dive
Big data little data is a fascinating interplay between massive datasets and smaller, focused ones. While big data often gets the spotlight for its potential, little data holds surprising power in specific contexts. This exploration delves into the unique characteristics, collection methods, and analysis techniques of both, highlighting how they can work together for comprehensive insights.
This post will examine the different types of little data, from customer feedback surveys to A/B testing results, showcasing their crucial role in understanding specific needs and tailoring solutions. We’ll also compare and contrast the characteristics of big and little data, exploring how they are collected, stored, and analyzed. Finally, we’ll touch on the challenges and ethical considerations of utilizing both data types, offering practical strategies to mitigate potential issues.
Defining Big Data and Little Data
Big data and little data are two sides of the same coin in the vast landscape of data analysis. While big data often gets the spotlight, understanding little data’s role is crucial for a comprehensive perspective. This exploration delves into the characteristics of each, their interconnectedness, and the different types of little data.The analysis of data, from massive datasets to minute details, is essential for effective decision-making.
Understanding the distinctions between big data and little data allows for more targeted and effective use of these data sources. This is not just about volume, but also about the context and application of the data.
Defining Big Data
Big data is characterized by its massive volume, high velocity, diverse variety, potentially questionable veracity, and the potential for significant value. This means it encompasses enormous amounts of data, often generated at rapid speeds, from various sources (structured, semi-structured, and unstructured). The reliability of the data can vary, requiring careful scrutiny and processing. However, the potential for deriving valuable insights from big data is substantial.
For example, social media feeds, sensor data from manufacturing processes, and online transaction records all contribute to the massive volume and variety of big data.
Defining Little Data
Little data, in contrast to big data, comprises relatively small datasets. These datasets are often focused on specific, niche areas and characterized by low velocity, high veracity, and substantial value within their context. The small size allows for deeper investigation and understanding, often leading to more accurate insights compared to the broader scope of big data. While the volume is less significant, the reliability and precision of little data are usually higher.
Relationship Between Big Data and Little Data
Big data and little data are not mutually exclusive; they are interconnected. Big data can be broken down into smaller, more manageable chunks, which are then analyzed using little data techniques. This approach allows for a more comprehensive understanding of the entire dataset. Conversely, little data insights can be used to enrich and contextualize big data, increasing the value derived from the analysis.
Big data, little data – it’s all about the insights, right? And those insights are crucial for firms like the ones listed in largest architectural firms 2. Knowing which projects to prioritize, materials to use, and how to manage resources efficiently all rely on a good understanding of both massive and smaller datasets. Ultimately, the key to success in any industry, from architecture to anything else, is effective data analysis, regardless of scale.
In effect, big data provides a broader view, while little data allows for a more nuanced understanding.
Types of Little Data
Little data comes in various forms, each with its own characteristics and applications.
- Customer Relationship Management (CRM) data: This data, often gathered through customer interactions, includes details like purchase history, demographics, and preferences. It is highly valuable for personalized marketing and targeted product recommendations.
- Internal company data: This data comes from within an organization, such as employee performance metrics, project progress reports, and departmental budgets. It’s critical for internal performance monitoring and decision-making.
- Expert opinions: This type of data includes insights from experienced professionals. It can be crucial for validating big data findings and offering specialized context. Examples include industry experts’ perspectives on market trends or engineers’ assessments of machine performance.
- Historical data: This can include records of past events, experiments, or customer interactions. It provides a baseline for comparison and trend analysis, particularly when combined with current data.
Comparison of Big Data and Little Data
The following table summarizes the key differences between big data and little data in terms of characteristics and applications.
Characteristic | Big Data | Little Data |
---|---|---|
Volume | Massive | Relatively small |
Velocity | High | Low |
Variety | Diverse | Specific |
Veracity | Potentially unreliable | More trustworthy |
Value | Potential for high value | High value in specific context |
Data Collection Methods for Big Data and Little Data: Big Data Little Data
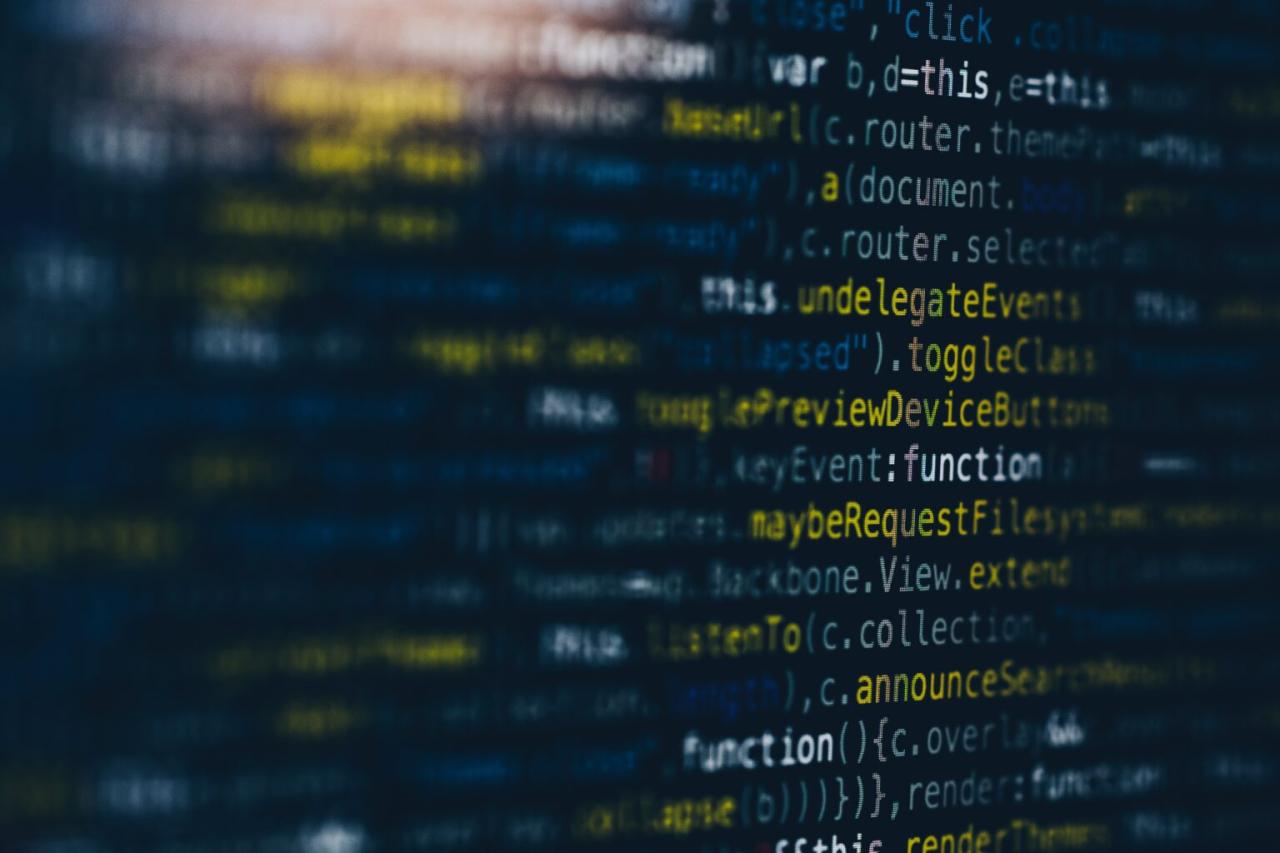
Data collection is the cornerstone of any data-driven endeavor. Understanding the nuances of how data is gathered is crucial for ensuring the quality and reliability of insights derived from it. This exploration dives into the methods employed for collecting both massive datasets (big data) and smaller, focused datasets (little data), highlighting the strengths and considerations for each approach.Data collection methods are intrinsically linked to the type of analysis desired.
Big data often necessitates methods that can handle the sheer volume and velocity of information, while little data demands precise and targeted approaches that maximize the value of each piece of information. This article details these methodologies, offering examples to illustrate their practical applications.
Data Collection Methods for Big Data
Big data often involves collecting information from diverse and numerous sources. The sheer volume and velocity of this data necessitate methods that can efficiently process and store it. Techniques like sensor networks and web scraping are crucial for gathering large quantities of data.
Big data, little data – it’s all about finding the insights, right? And sometimes, those insights are as big as a new river cruise ship! Just like the recent christening of two new vessels by Avalon, avalon christens two river cruise ships , the sheer volume of passenger data they gather could be considered big data, even if the individual passenger records seem small.
Ultimately, both big and little data contribute to the whole picture, shaping the future of travel and, perhaps, even the future of data analysis itself.
- Sensor Networks: These networks use interconnected sensors to collect data from various physical environments. Examples include monitoring environmental conditions (temperature, humidity, air quality), tracking vehicle movement, or monitoring industrial processes. Considerations for sensor networks include sensor accuracy, network connectivity, and data transmission protocols.
- Web Scraping: This automated method extracts data from websites. It’s valuable for gathering structured data from online sources, such as product listings, news articles, or social media posts. Ethical considerations and website terms of service must be respected when using web scraping.
- Machine Learning-Based Data Collection: In some cases, machine learning algorithms can identify and collect relevant data automatically. For example, an algorithm trained on user behavior can automatically collect data from various sources relevant to a specific task, such as identifying potential customer churn.
Data Collection Methods for Little Data
Little data, characterized by its focused nature, necessitates methods that ensure accuracy and efficiency. Methods like surveys, interviews, and experiments provide valuable insights into specific populations or phenomena.
- Surveys: Surveys gather data through structured questionnaires administered to a targeted group. These can be online, paper-based, or conducted in person. The key to successful surveys lies in the careful design of questions to elicit accurate and meaningful responses. Proper sampling techniques are crucial to ensure the survey accurately reflects the target population.
- Interviews: In-depth interviews provide qualitative data through conversations with individuals. These interviews allow for a deeper understanding of motivations, perspectives, and experiences. Careful planning, including structuring the interview questions, is essential to maximize the insights gained.
- Experiments: Experiments involve manipulating variables to observe their effect on a specific outcome. A/B testing, for instance, compares two versions of a product or service to determine which performs better. The careful control of variables in experiments is crucial to isolate the impact of the manipulated factors.
Data Collection Strategies for Big and Little Data
The following table Artikels different data collection strategies for both big and little data.
Data Type | Collection Method | Example |
---|---|---|
Big Data | Sensor networks, web scraping, machine learning-based collection | Tracking user behavior on a website, monitoring IoT devices, collecting stock market data |
Little Data | Surveys, interviews, experiments, focus groups | Gathering customer feedback through surveys, conducting A/B testing, conducting user interviews |
Data Storage and Management
Storing and managing data, whether it’s a few gigabytes or petabytes, is crucial for effective analysis and decision-making. The methods used for big data and little data differ significantly due to the volume and velocity of the data itself. Effective strategies ensure data accessibility, security, and efficient retrieval for analysis.The diverse nature of data requires a tailored approach to storage and management.
Big data, little data – it’s all about finding the nuggets of gold in the mountain of information, right? That’s definitely a useful concept to consider when planning your next trip, like the Avalon Alegria’s first call, avalon alegria first call. Ultimately, whether it’s a massive dataset or a smaller one, effective analysis and strategic decision-making are key to making the most of any trip, or in any big data, little data scenario.
This encompasses understanding the specific characteristics of the data—its structure, format, and expected usage—to select the appropriate storage solution.
Big Data Storage Solutions
Different big data storage solutions cater to the unique needs of massive datasets. Choosing the right solution depends on factors such as scalability, performance, and cost-effectiveness. Distributed file systems, such as Hadoop Distributed File System (HDFS), are designed for handling vast amounts of data across multiple servers. This distributed architecture allows for high scalability, making it ideal for petabytes of data.
Big data and little data – it’s all about perspective, isn’t it? Just like how a seemingly simple journey can be deeply enriching when you trace its roots back to its origins, an exceptional tour traced to its roots like this one can reveal fascinating stories. Ultimately, the journey of discovering those details, whether in vast datasets or small samples, offers unique insights and a richer understanding of the subject.
The more we delve into the “little data” the more we appreciate the bigger picture. It’s all connected.
NoSQL databases, such as MongoDB, offer flexible schemas, accommodating unstructured or semi-structured data often found in big data environments. Cloud-based storage solutions, like Amazon S3, provide scalability on demand and are cost-effective for large datasets.
Big Data Management Strategies
Effective big data management necessitates strategies to handle data’s complexity. Data warehousing and data lakes are crucial for storing and organizing large datasets. Data warehousing involves structured data, while data lakes store both structured and unstructured data. Data pipelines are essential for moving data between storage systems and processing platforms, ensuring data availability for analysis. Data governance policies and procedures are critical for ensuring data quality, security, and compliance with regulations.
These policies should cover access controls, data validation, and data retention. Data encryption and access controls are fundamental for maintaining security and privacy.
Little Data Storage and Management
Managing little data requires simpler approaches compared to big data. Traditional relational databases, like MySQL or PostgreSQL, are suitable for structured little data. These systems offer robust query capabilities and data integrity. File systems, like local file storage or cloud storage for smaller files, can effectively handle little data. Choosing the right database or file system is essential for ensuring data integrity and accessibility.
Data Organization for Analysis
Effective data organization is crucial for successful analysis. Data should be structured logically to facilitate queries and analysis. Schema design and data modeling play a significant role in achieving this. Data normalization, which reduces data redundancy and improves data integrity, is an essential technique for structured data. Metadata management is crucial for understanding data characteristics, origin, and quality, which is vital for accurate analysis.
Big data, little data – it’s all about perspective, right? Thinking about how much data is involved in showcasing the incredible talent at the academy kicks off 58th artists of hawaii exhibit , it’s fascinating how even a seemingly small dataset can be incredibly valuable. From artist biographies to exhibit attendance, a thoughtful collection of information can reveal insights into artistic trends and public response, demonstrating the power of big data, even in small packages.
Little Data Organization and Management Process
Organizing and managing little data involves a structured process. This includes data validation to ensure data quality. Establish clear data naming conventions and document data sources for easy tracking and understanding. Data cleansing is crucial to eliminate inconsistencies and errors, ensuring accurate analysis. Regular backups are vital to prevent data loss.
Data documentation is key for long-term maintenance and analysis.
Analysis Techniques
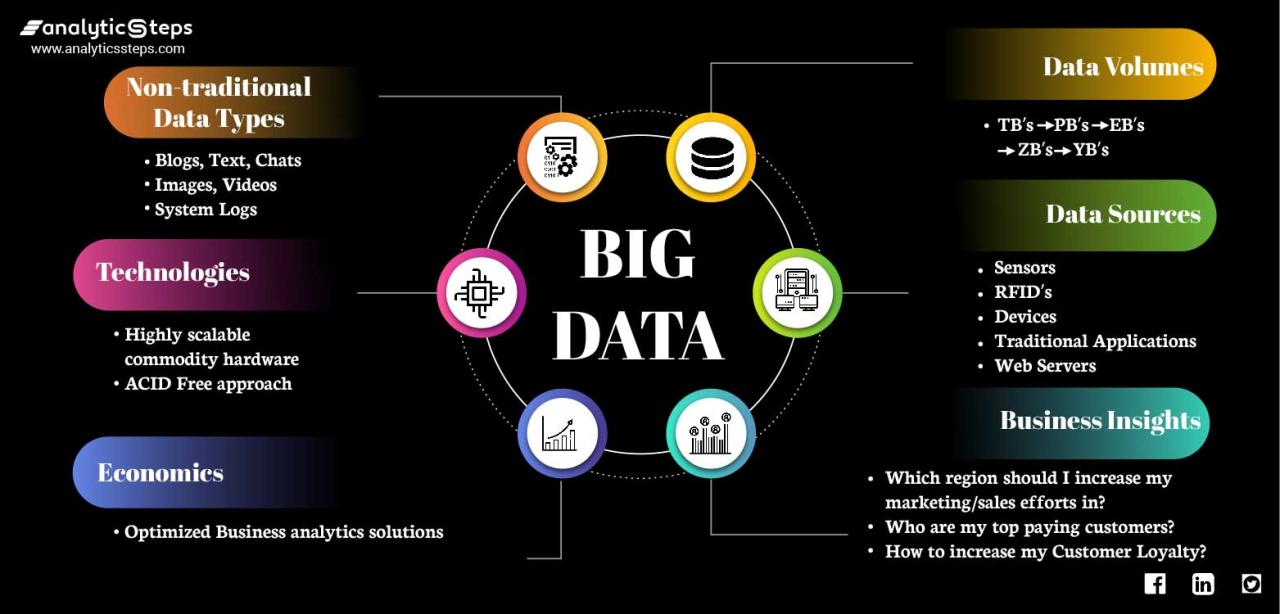
Analyzing data, whether a massive dataset or a small sample, is crucial for extracting meaningful insights. Different analytical techniques are employed depending on the data’s volume and characteristics. This section delves into the contrasting approaches for big and little data, highlighting the statistical and machine learning methods suitable for each.Analytical techniques must be tailored to the specific nature of the data.
For big data, the focus is on scalability and efficiency, while little data analysis emphasizes the use of sophisticated statistical methods to extract the most valuable information from the limited sample. Understanding the strengths and weaknesses of each approach is vital for effective data interpretation.
Analytical Techniques for Big Data
Big data analysis necessitates techniques that can handle massive volumes of data efficiently. Distributed computing frameworks like Hadoop and Spark are commonly used to process large datasets in parallel. These frameworks enable the application of algorithms across multiple machines, accelerating the analysis process. Specific techniques include:
- Clustering Algorithms: Algorithms like K-means and DBSCAN group similar data points together, useful for tasks like customer segmentation or identifying patterns in large datasets.
- Association Rule Mining: Methods like Apriori and FP-growth identify relationships between variables within massive datasets, enabling insights into customer purchase patterns or product recommendations.
- Predictive Modeling: Big data is ideal for building complex predictive models. These models, often using machine learning techniques, forecast future trends or outcomes. Examples include fraud detection in financial transactions or predicting equipment failures.
Analytical Techniques for Little Data
Little data, while less voluminous, requires different analytical techniques that focus on extracting the maximum possible information from the limited sample. The focus is on detailed understanding and robust statistical analysis. Key techniques include:
- Descriptive Statistics: Measures of central tendency (mean, median, mode) and dispersion (standard deviation, variance) provide a comprehensive summary of the data. For instance, these measures can highlight the typical characteristics of a product’s performance.
- Inferential Statistics: These techniques use statistical tests (t-tests, ANOVA) to make inferences about a population based on a sample. For example, inferential statistics help determine if there is a statistically significant difference between two groups.
- Regression Analysis: Linear and non-linear regression models can identify relationships between variables, crucial for forecasting or understanding cause-and-effect relationships. An example is analyzing how advertising spending impacts sales.
Machine Learning for Big and Little Data
Machine learning (ML) algorithms can be applied to both big and little data. ML models learn from data to identify patterns and make predictions. The choice of model depends on the nature of the data and the desired outcome.
- Big Data: ML algorithms like neural networks, support vector machines, and random forests are well-suited for big data. Their ability to handle complex relationships in massive datasets is a significant advantage. However, training these models on massive datasets can be computationally expensive and require significant resources.
- Little Data: For little data, simpler ML models like logistic regression, naive Bayes, and decision trees are often preferred. These models are less computationally intensive and can provide valuable insights with limited data. However, their performance can be affected by overfitting to the small dataset, so careful consideration of model complexity is essential.
Specific Machine Learning Models for Little Data
Choosing the right ML model for little data requires careful consideration. Overfitting is a major concern, as a model trained on a small dataset may not generalize well to new data. Specific models suitable for little data include:
- Logistic Regression: A linear model for binary classification problems. Its simplicity and interpretability make it suitable for little data analysis.
- Naive Bayes: A probabilistic classifier based on Bayes’ theorem. Its efficiency and ability to handle high-dimensional data make it a good choice for limited data sets.
- Support Vector Machines (SVMs): Models that find optimal hyperplanes to separate different classes. SVMs can be effective with little data, but the choice of kernel function is crucial for optimal performance.
Applications and Use Cases
Big data and little data, despite their contrasting natures, are powerful tools when used strategically. Understanding their applications and use cases across diverse industries reveals their potential to drive innovation and decision-making. The combination of these two data types offers a more comprehensive understanding than either could achieve alone.Combining massive datasets with smaller, focused ones can lead to more accurate and nuanced insights.
This synergy allows organizations to uncover hidden patterns, personalize experiences, and optimize processes in ways previously unimaginable. Real-world examples demonstrate the transformative impact of big data and little data, and their successful integration.
Real-World Applications of Big Data
Big data’s vast scope allows for analysis across numerous industries, enabling businesses to make informed decisions based on detailed insights. Retailers, for example, can track consumer preferences, buying patterns, and demographics to personalize marketing strategies and optimize inventory management. Predictive analytics based on massive datasets helps anticipate demand fluctuations and minimize stockouts. This allows for a more efficient supply chain and enhanced customer satisfaction.
- Healthcare: Analyzing patient records, medical images, and research data helps identify trends in diseases, predict outbreaks, and develop personalized treatment plans. This can improve diagnosis accuracy and patient outcomes.
- Finance: Financial institutions leverage big data to detect fraudulent transactions, assess credit risk, and personalize financial products. Algorithms analyze vast datasets of transactions and market data to identify patterns and anomalies, minimizing financial losses.
- Transportation: Big data analysis of traffic patterns, vehicle performance, and road conditions helps optimize transportation networks. This leads to reduced congestion, improved fuel efficiency, and enhanced safety.
Practical Examples of Little Data Applications
Little data, while not possessing the same scale as big data, is invaluable in specific contexts. For example, in niche markets or specialized industries, detailed insights from focused datasets can provide a significant advantage.
- Small Businesses: A small bakery can track customer preferences through direct interaction and surveys, enabling them to tailor their offerings and optimize their production. Detailed feedback on customer satisfaction and product preferences, even with limited data, allows them to personalize their services.
- Local Governments: A city can gather data on local crime patterns, traffic flow, and environmental conditions to improve public safety and infrastructure. These insights from focused areas allow them to effectively allocate resources and address community needs more precisely.
Big Data and Little Data in Conjunction, Big data little data
Big data and little data can be exceptionally powerful when combined. The vast scope of big data can provide context and scale, while little data offers precision and nuanced understanding.
- Personalized Recommendations: A streaming service can use big data to identify popular content and trends, then use little data from user interactions (e.g., ratings, comments, watch history) to personalize recommendations. This combination yields more accurate and engaging suggestions than relying on big data alone.
- Customer Relationship Management (CRM): Companies can use big data to understand overall customer behavior and trends. Little data, from individual customer interactions and feedback, can provide a deeper understanding of their specific needs and preferences. This allows businesses to tailor their products and services to each customer, maximizing satisfaction.
Industries Utilizing Big Data and Little Data
Numerous industries leverage both big data and little data for improved decision-making and enhanced customer experience.
Industry | Big Data Application | Little Data Application |
---|---|---|
Retail | Predictive analytics for inventory management, personalized marketing | Customer feedback surveys, loyalty program data |
Healthcare | Disease prediction, personalized treatment plans | Patient medical history, doctor-patient interactions |
Finance | Fraud detection, risk assessment | Customer financial transactions, loan applications |
Challenges and Considerations
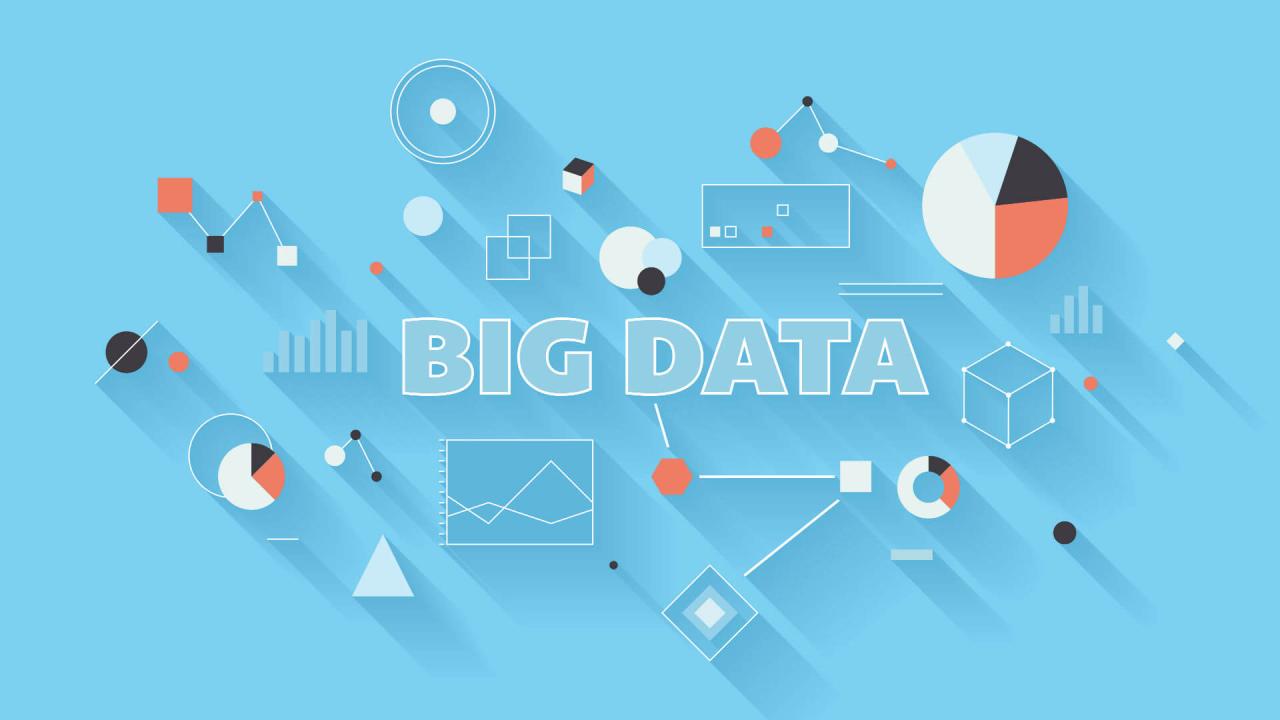
Navigating the landscapes of big and little data presents unique hurdles. While the potential benefits are immense, the practical application often faces obstacles related to data volume, quality, and the specific analytical requirements. Addressing these challenges is crucial for extracting meaningful insights and avoiding pitfalls in decision-making.Data handling, whether in vast quantities or limited samples, demands careful consideration of potential biases and limitations.
Strategies for mitigating these issues and ensuring ethical use are paramount to responsible data science practices.
Challenges of Big Data
The sheer volume, velocity, and variety of big data often present significant challenges. Managing and processing this massive influx of information requires sophisticated infrastructure and specialized tools. Data storage and retrieval are critical concerns, as are the computational resources needed for complex analyses. Additionally, the quality of data can vary, introducing inconsistencies and inaccuracies that affect the reliability of insights.
- Storage and Infrastructure: Storing petabytes of data demands robust and scalable storage solutions. Traditional database systems often struggle with the volume and velocity of big data. Cloud-based solutions offer scalable storage and processing capabilities, but managing data security and privacy in these environments requires careful planning and implementation.
- Processing Power: Analyzing big data requires significant computational power. Complex algorithms and parallel processing techniques are essential to extract meaningful patterns and insights from vast datasets. The cost of computing resources can be substantial, especially for large-scale projects.
- Data Quality and Cleaning: Big datasets often contain errors, inconsistencies, and missing values. Data cleaning and preprocessing steps are essential to ensure the accuracy and reliability of analyses. The time and resources dedicated to data cleaning can be substantial.
Challenges of Little Data
Working with limited datasets presents its own set of challenges. The primary concern is the potential for insufficient sample size to accurately represent the population. This can lead to biased results and inaccurate conclusions. Furthermore, limited data may hinder the application of complex analytical techniques, restricting the scope of possible discoveries.
- Sample Size and Representativeness: Small sample sizes can lead to unreliable estimations and projections. A limited sample might not adequately reflect the diversity of the population, potentially leading to inaccurate conclusions. Statistical techniques to account for limited data are crucial to make informed decisions based on limited samples.
- Generalizability of Results: Insights drawn from little data may not be generalizable to the broader population. The limited scope of the sample can hinder the ability to extrapolate findings to other settings or contexts.
- Limited Analytical Options: Complex analytical techniques often require a certain minimum dataset size. With little data, the choice of analytical methods is often restricted, limiting the potential depth of insights.
Biases and Limitations
Both big data and little data can be susceptible to various biases. In big data, biases can stem from data collection methods, representation of subgroups, or inherent inaccuracies within the data itself. In little data, biases can arise from sampling methods or the inherent limitations of the sample size.
- Selection Bias: Data collection methods can introduce biases. For example, if a survey only targets a specific demographic, the results may not accurately reflect the broader population. This is equally true for big data, where skewed data collection can lead to inaccurate representation.
- Measurement Error: Inaccurate measurements or poorly defined variables can introduce errors in both big data and little data analysis. Careful consideration of measurement methodologies is vital to minimize these errors.
- Confirmation Bias: The tendency to seek or interpret information that confirms existing beliefs can bias the analysis of both big and little data. Critical evaluation of assumptions and potential biases is paramount.
Mitigation Strategies
Addressing the challenges associated with big and little data requires proactive strategies. These include employing robust data collection methods, implementing quality control measures, and utilizing appropriate analytical techniques.
- Data Validation and Quality Control: Rigorous data validation procedures can help identify and correct errors and inconsistencies in both big and little data sets. This process helps improve data reliability and accuracy.
- Sampling Techniques: For little data, employing appropriate sampling techniques is essential. Strategies like stratified sampling can help ensure that the sample accurately reflects the population’s characteristics. Big data may require sampling to manage volume.
- Robust Statistical Methods: Appropriate statistical methods can help mitigate the effects of limited sample sizes or biases in little data analysis. For big data, advanced algorithms and techniques are needed to process and extract valuable information.
Ethical Considerations
The ethical implications of big and little data use are substantial. Issues of privacy, fairness, and accountability must be carefully considered. Data security and responsible use are crucial to prevent misuse or unintended consequences.
- Data Privacy: Protecting individual privacy is paramount when handling personal data, regardless of dataset size. Data anonymization and appropriate data security measures are essential to prevent misuse and unauthorized access.
- Algorithmic Bias: Algorithms trained on biased data can perpetuate or amplify existing societal inequalities. Careful consideration of potential biases and the fairness of algorithms is crucial.
- Transparency and Accountability: Data analysis should be transparent, and the decision-making processes should be accountable. Clear communication and documentation of methods and results are essential for ethical data use.
Closing Notes
In conclusion, big data and little data, though seemingly disparate, are complementary forces. Big data provides the broad strokes, revealing patterns and trends across vast populations, while little data offers the granular details needed to tailor solutions and personalize experiences. Understanding their individual strengths and how they can be integrated is crucial for unlocking their full potential. By leveraging both, businesses and organizations can achieve deeper insights, more targeted strategies, and ultimately, more impactful results.
FAQ Compilation
What are some examples of little data sources?
Little data sources can include surveys, interviews, focus groups, A/B testing results, and customer feedback forms. These often provide in-depth insights into specific user behaviors or preferences.
How can big and little data be combined for better insights?
Combining big data’s broad trends with little data’s detailed specifics allows for a more comprehensive understanding. For example, big data might reveal a general preference for a product feature, while little data can pinpoint which specific segment of users are most passionate about that feature.
What are the ethical implications of using big data and little data?
Ethical concerns surrounding both big and little data include privacy, bias, and potential misuse. Careful consideration of these factors is crucial to ensure responsible data collection, analysis, and application.
What are some common biases in data analysis?
Biases can arise from various sources, including sample selection, data collection methods, and the analysis techniques themselves. Understanding and mitigating potential biases is vital for drawing accurate conclusions from both big and little data.