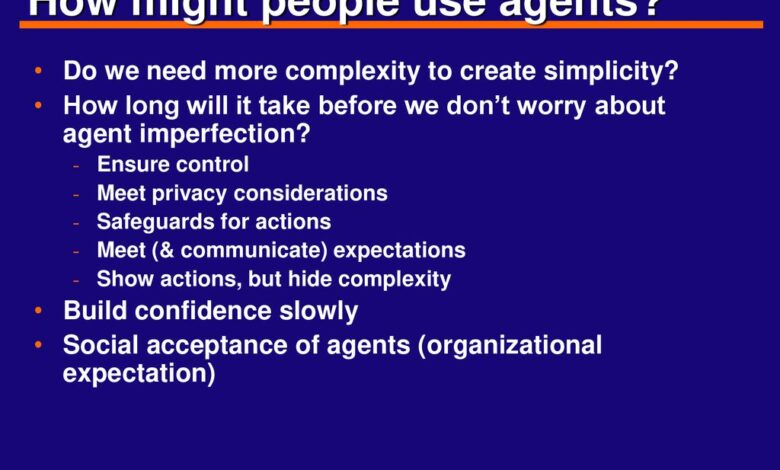
Agents Must Harness the Power of Data
Agents must harness the power of data to thrive in today’s dynamic world. Data-driven decision-making is no longer a luxury, but a necessity for success. From understanding market trends to anticipating customer behavior, data provides agents with invaluable insights to optimize their strategies and achieve remarkable results. This exploration dives deep into how agents can leverage data effectively across various aspects of their operations.
This post will explore the crucial role of data in modern decision-making processes for agents. We will cover everything from data collection and management to analysis and interpretation, and ultimately, how to translate those insights into actionable strategies for improvement. Real-world case studies and future trends will be discussed, providing a comprehensive guide for agents looking to maximize their impact through data.
Data-Driven Decision Making
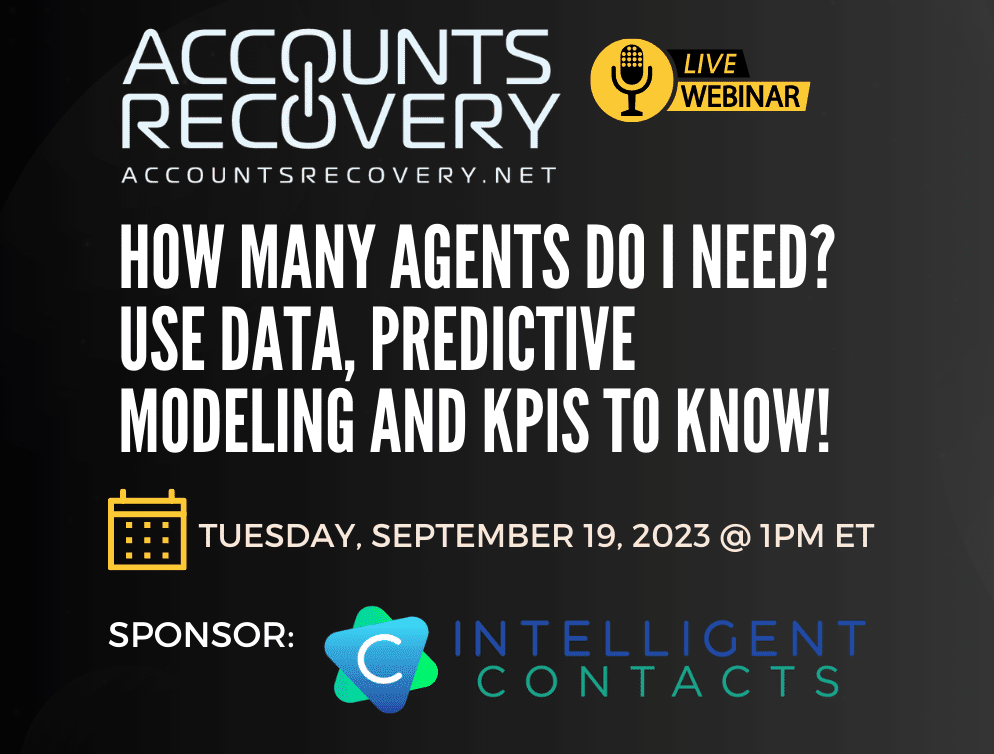
Data has become the lifeblood of modern operations, influencing everything from strategic choices to everyday tasks. Agents in various sectors, from finance to healthcare, are recognizing the profound impact of data-driven decision making. Leveraging data allows agents to make informed choices, optimize processes, and anticipate future trends. This approach empowers agents to act proactively rather than reactively, fostering greater efficiency and profitability.Data analysis, when properly implemented, is no longer a luxury but a necessity for agents seeking a competitive edge.
It provides a comprehensive view of performance, customer behavior, and market trends, ultimately shaping the trajectory of an organization. This understanding allows agents to make calculated risks and allocate resources effectively. Data-driven insights help agents move beyond assumptions and embrace concrete evidence in their decision-making processes.
The Role of Data in Strategic Choices
Data plays a crucial role in shaping strategic choices for agents. By analyzing market trends, customer preferences, and operational metrics, agents can identify opportunities and mitigate risks. This analysis enables the development of tailored strategies that are more likely to succeed. Data allows agents to adapt to changing conditions, ensuring that their strategies remain aligned with current realities.
For instance, an agent in the retail sector can use data to understand consumer buying patterns and adjust inventory accordingly.
Agents need to embrace data analysis to stay competitive, especially now that Mondovi will soon be under Emplify Health, a significant development in the industry. Understanding customer trends and market shifts through data is crucial for success. By leveraging data insights, agents can anticipate needs and offer tailored solutions, ultimately boosting their performance in this evolving landscape.
Examples of Successful Data-Driven Strategies
Several agents have successfully implemented data-driven strategies across various sectors. In the financial industry, quantitative analysis is routinely used to predict market movements and make investment decisions. Retailers leverage customer purchase history to personalize recommendations and tailor marketing campaigns. In healthcare, data analysis is used to improve patient outcomes and optimize resource allocation. These examples highlight the versatility and impact of data-driven strategies.
Predicting Future Trends and Outcomes
Data analysis allows agents to predict future trends and outcomes. By identifying patterns and correlations in historical data, agents can anticipate changes in the market, customer behavior, and operational efficiency. For example, an e-commerce company can use data on past sales trends and website traffic to predict future demand and adjust inventory levels accordingly. Analyzing social media sentiment can provide valuable insights into public opinion and emerging trends, enabling proactive responses.
The ability to anticipate future developments is crucial for agents to maintain a competitive edge.
Types of Data and Their Applications
Data Type | Description | Application |
---|---|---|
Market Trends | Information about market conditions, including pricing, demand, and supply. | Identifying new market opportunities, adjusting pricing strategies, and anticipating future market fluctuations. |
Customer Behavior | Data about customer preferences, purchasing habits, and interactions with the agent. | Personalizing marketing campaigns, improving customer service, and developing new products and services. |
Operational Metrics | Data related to internal processes, such as efficiency, productivity, and cost. | Identifying areas for improvement, optimizing resource allocation, and streamlining operations. |
Competitor Analysis | Information about competitors’ strategies, performance, and market share. | Developing strategies to gain a competitive edge, identifying weaknesses in competitors’ strategies, and anticipating competitor actions. |
Data analysis enables agents to make well-informed decisions that align with their goals and objectives. This table demonstrates how diverse data sources can be used to enhance strategic planning and achieve optimal outcomes.
Data Collection and Management
Effective data collection and management are critical for agents to make informed decisions and provide exceptional service. A well-structured data strategy ensures that the right information is gathered, stored, and utilized, leading to improved efficiency and better outcomes. This approach also fosters trust and transparency with clients.Data collection is not just about gathering information; it’s about strategically collecting the precise data needed to solve problems and enhance customer experiences.
This involves identifying the key performance indicators (KPIs) and metrics relevant to agent tasks and client interactions. A robust data management system ensures these metrics are tracked consistently and accurately.
Efficient Data Collection Strategies
A well-defined data collection strategy ensures that the right data is gathered in the right way. This includes identifying the necessary data points, selecting appropriate collection methods (e.g., surveys, forms, observations), and establishing clear procedures to ensure data accuracy and consistency. Thorough data collection procedures help agents understand client needs better and make more effective decisions.
- Defining Data Requirements: Clear identification of the specific data needed for analysis and decision-making is essential. This includes determining the source of data, the frequency of collection, and the format of the data.
- Choosing Appropriate Collection Methods: Selection of appropriate methods based on the nature of the data and the resources available. This could involve using online forms, questionnaires, automated data capture systems, or manual data entry.
- Ensuring Data Quality: Implementing measures to maintain data accuracy, consistency, and completeness is crucial. This includes validation checks, data cleaning procedures, and regular data audits.
Organizing and Storing Diverse Data Sets
Effective organization and storage of diverse data sets is essential for efficient retrieval and analysis. This involves implementing a structured approach to data categorization, classification, and storage. Choosing the right storage methods ensures accessibility and reduces redundancy.
- Data Categorization and Classification: Developing a clear system for classifying data based on relevant criteria (e.g., client type, product category, interaction type). This structured approach facilitates easier retrieval and analysis.
- Data Storage Formats: Selecting appropriate storage formats that support efficient retrieval and analysis. Examples include relational databases for structured data, NoSQL databases for unstructured data, and cloud storage for scalable storage.
- Data Backup and Recovery: Implementing a robust data backup and recovery plan to protect against data loss due to system failures, human error, or malicious attacks. This ensures business continuity and prevents significant disruptions.
Data Security and Privacy, Agents must harness the power of data
Data security and privacy are paramount. Implementing strong security measures to protect sensitive data from unauthorized access, use, or disclosure is critical. This includes complying with relevant data privacy regulations.
- Data Encryption: Protecting sensitive data by encrypting it both in transit and at rest. This ensures that only authorized personnel can access the data.
- Access Control: Implementing strict access controls to limit data access to only authorized personnel. This includes defining user roles and permissions to manage data access effectively.
- Compliance with Regulations: Adhering to relevant data privacy regulations (e.g., GDPR, CCPA) to ensure compliance and maintain client trust. This includes implementing data protection policies and procedures.
Tools and Technologies for Effective Data Management
A variety of tools and technologies can support effective data management for agents. These tools help with data collection, storage, and analysis.
- Database Management Systems (DBMS): Relational databases (like MySQL, PostgreSQL) and NoSQL databases (like MongoDB, Cassandra) are crucial for structured and unstructured data storage, respectively.
- Cloud Storage Solutions: Cloud providers (like Amazon S3, Google Cloud Storage) offer scalable and cost-effective storage solutions for large datasets.
- Data Visualization Tools: Tools like Tableau, Power BI, and Qlik Sense help visualize data trends and patterns for better understanding.
Data Storage Solutions Comparison
The choice of data storage solution depends on specific needs and requirements.
Data Storage Solution | Strengths | Weaknesses |
---|---|---|
Cloud Storage (e.g., Amazon S3) | Scalability, cost-effectiveness, accessibility, and high availability. | Potential security concerns if not properly configured, vendor lock-in, and limited control over infrastructure. |
Relational Databases (e.g., MySQL) | Structured data management, strong querying capabilities, ACID properties, and data integrity. | Can be less flexible for unstructured data, potentially higher costs for very large datasets, and less scalable. |
NoSQL Databases (e.g., MongoDB) | Scalability, flexibility, and handling of unstructured data. | Potential complexity in querying, lack of ACID properties, and potentially less data integrity. |
Data Analysis and Interpretation
Unleashing the true potential of data requires more than just collection and management; it demands insightful analysis and interpretation. This crucial step transforms raw data into actionable intelligence, empowering agents to make informed decisions and drive impactful results. Data analysis techniques provide the tools to extract meaningful patterns, trends, and relationships from the collected information. Algorithms and models play a vital role in this process, enabling agents to uncover hidden insights and predict future outcomes.
Effective visualizations further enhance understanding, ensuring that the insights are readily accessible and comprehensible.
Agents absolutely must harness the power of data to stay competitive. This is crucial for understanding market trends and making informed decisions, especially in the ever-evolving field of architectural design. For example, looking at the strategies employed by the largest architectural firms, like those detailed in this piece on largest architectural firms 2 , reveals how data-driven insights can shape successful projects.
Ultimately, successful agents will be those who best utilize data to gain a competitive edge.
Data Analysis Techniques
Data analysis encompasses a wide array of techniques, each suited to specific situations and types of data. Understanding these techniques is crucial for agents to choose the most appropriate method for their needs. A diverse toolkit allows agents to analyze data effectively.
- Statistical analysis employs mathematical methods to identify patterns and relationships in data. Techniques like regression analysis, hypothesis testing, and correlation analysis help uncover trends and dependencies within the dataset. For example, statistical analysis can be used to determine the relationship between marketing spend and sales revenue.
- Machine learning algorithms, on the other hand, allow agents to identify complex patterns and relationships in data without explicit programming. Supervised learning techniques, like classification and regression, enable agents to build models that predict future outcomes based on past data. Unsupervised learning methods, like clustering and dimensionality reduction, help discover hidden structures and relationships in data. For instance, machine learning algorithms can predict customer churn based on historical data.
- Text analysis, a specialized technique, focuses on extracting insights from textual data. Natural language processing (NLP) methods are used to analyze documents, social media posts, and other textual data to understand sentiment, identify s, and extract relevant information. For instance, text analysis can be used to understand customer feedback and identify areas for improvement.
Role of Algorithms and Models
Algorithms and models are fundamental tools in data interpretation, enabling agents to automate the analysis process and identify hidden patterns. They provide a structured approach to extracting insights from complex data sets.
- Regression models, for instance, can be used to predict the future value of a variable based on the values of other variables. Linear regression models establish a relationship between variables through a straight line. Other models, such as polynomial regression, may fit a curved relationship between variables. For example, a company might use a regression model to predict future sales based on historical sales data, advertising spend, and economic indicators.
- Classification algorithms categorize data into predefined groups. Support vector machines (SVMs) and decision trees are common examples. These models are useful in identifying patterns and predicting outcomes. For example, a financial institution can use classification models to categorize loan applications as high-risk or low-risk.
Data Visualizations
Effective visualization techniques are crucial for communicating insights to agents. Visual representations transform complex data into easily understandable formats, facilitating quicker comprehension and faster decision-making.
- Bar charts and line graphs are simple yet powerful tools for displaying trends and comparisons. For example, a bar chart can be used to display sales figures for different product categories over a period. A line graph can illustrate the growth of a company’s revenue over time.
- Scatter plots visually represent the relationship between two variables. They can reveal correlations and patterns in the data. For instance, a scatter plot can be used to show the relationship between customer age and spending habits.
- Heatmaps and treemaps are used to display data in a visually engaging manner. They provide a quick overview of large datasets and highlight important trends. For example, a heatmap can illustrate the popularity of different products in different regions.
Data Interpretation and Decision-Making
Data interpretation is the bridge between raw data and informed decisions. It enables agents to extract meaningful insights from the data and apply them to their work.
Travel agents need to embrace data-driven strategies. For example, understanding customer preferences can be key to crafting personalized itineraries, like a bite size sailing experience, a bite size sailing experience. Ultimately, this means leveraging data to tailor experiences and stay ahead of the curve in the competitive travel industry.
- Data interpretation empowers agents to understand patterns and trends in the data, leading to more strategic decisions. For example, an e-commerce company might notice a surge in sales of a particular product during a specific time of year. By interpreting this data, they can adjust inventory levels, marketing campaigns, and pricing strategies accordingly.
Comparison of Data Analysis Methods
Analysis Method | Description | Suitability |
---|---|---|
Statistical Analysis | Uses mathematical methods to identify patterns and relationships in data. | Suitable for understanding relationships between variables, identifying trends, and testing hypotheses. |
Machine Learning | Employs algorithms to discover patterns and make predictions. | Suitable for complex data sets, identifying hidden patterns, and predicting future outcomes. |
Text Analysis | Focuses on extracting insights from textual data. | Suitable for understanding customer feedback, sentiment analysis, and identifying s. |
Data-Powered Action and Improvement
Data isn’t just for analysis; it’s a catalyst for change. Transforming insights into tangible improvements requires a strategic approach. This section delves into the practical application of data-driven decision-making, emphasizing the crucial role of feedback loops and actionable strategies for agents. We’ll explore how agents can leverage data to optimize their performance and efficiency, illustrated with real-world examples.Data-driven strategies aren’t static; they require ongoing refinement and adaptation.
Feedback loops are essential to identify areas for improvement and ensure that the data-driven approach remains effective and relevant. By continually assessing the impact of implemented strategies and gathering new data, agents can build a dynamic process for optimization.
Agents need to seriously embrace data analysis. It’s crucial for success in today’s fast-paced world. Seeing the dozens of graduates honored at the transformational leadership ceremony ( dozens of graduates honored at transformational leadership ceremony ) highlights the importance of strategic thinking and impactful leadership, which directly correlates with using data to inform decisions. Ultimately, mastering data is key to unlocking opportunities for agents everywhere.
Translating Data Insights into Actionable Strategies
Effective strategies emerge from clear connections between data insights and specific actions. Agents need to translate data-driven insights into actionable steps that directly address identified problems or opportunities. This involves defining measurable objectives, developing concrete plans, and allocating resources accordingly. For instance, if data reveals a significant drop in customer satisfaction related to a specific service, agents can develop targeted training programs or process improvements to address the issue.
The Importance of Feedback Loops
Feedback loops are critical for continuous improvement. They involve monitoring the effects of implemented strategies, gathering new data, and iterating on approaches based on observed results. A well-designed feedback loop will allow agents to identify areas where strategies are successful, where adjustments are needed, and what new data points are necessary to refine the strategy. This cyclical process ensures that data-driven approaches remain relevant and effective.
A key component is identifying metrics that track the impact of interventions and then adjusting the strategies based on this feedback.
Optimizing Performance and Efficiency with Data
Data empowers agents to identify areas for improvement and optimize their performance. By analyzing data on task completion times, error rates, and resource utilization, agents can pinpoint bottlenecks and inefficiencies. For example, if data indicates a high error rate in a particular task, agents can implement training programs to improve accuracy. If data reveals that certain tasks are taking longer than expected, agents can look at streamlining the processes.
Examples of Data-Driven Process Improvements
Numerous examples demonstrate the power of data-driven improvements. One company, for instance, used data to identify a bottleneck in its customer service queue. By analyzing call durations and customer feedback, they determined that a particular type of technical support query was significantly longer than average. They then implemented a self-service portal for these specific queries, reducing wait times and improving customer satisfaction.
Another company improved order fulfillment times by analyzing data on order processing steps and identifying inefficiencies in their warehouse operations. This led to the implementation of new software that automated several tasks, reducing errors and significantly speeding up delivery.
Step-by-Step Process for Data-Driven Improvements
Step | Description |
---|---|
1. Define Objectives | Clearly state the goals of the improvement initiative. |
2. Data Collection | Gather relevant data using appropriate tools and methods. Consider historical data and data from current operations. |
3. Data Analysis | Identify patterns, trends, and insights from the collected data. Use statistical analysis and visualization tools to understand the data effectively. |
4. Strategy Development | Formulate specific and actionable strategies based on the data insights. |
5. Implementation | Put the developed strategies into action. |
6. Monitoring and Evaluation | Continuously track the impact of implemented strategies. Collect data on the effectiveness of the changes and identify areas for improvement. |
7. Iteration | Use feedback to refine and improve the strategies, ensuring that data-driven improvements are sustained and optimized. |
Challenges and Considerations
Data, when wielded effectively, can be a powerful tool for agents, empowering them to make informed decisions and improve their performance. However, the path to data-driven success is rarely straightforward. Navigating the challenges and understanding the necessary considerations is crucial for any agent looking to fully harness the power of data. This section explores the obstacles, highlighting the importance of data quality, integration, skilled personnel, and proactive risk mitigation.
Potential Obstacles in Harnessing Data
Implementing a data-driven approach requires careful consideration of potential obstacles. These obstacles range from ensuring data quality and accuracy to managing diverse data sources and building a skilled team to utilize the data effectively. Addressing these challenges head-on is essential for successful data-driven operations.
- Data Quality and Accuracy: Inaccurate or incomplete data can lead to flawed analyses and ultimately poor decisions. Ensuring data accuracy requires rigorous validation processes, data cleansing procedures, and establishing clear data governance protocols. For example, if a sales agent relies on outdated customer data, they might miss crucial opportunities or make incorrect product recommendations. This emphasizes the importance of regular data updates and verification.
- Data Integration Challenges: Modern agents often work with data from various sources – CRM systems, marketing platforms, social media, and more. Integrating these disparate data sources into a unified platform can be complex and time-consuming. Data silos and inconsistent data formats can lead to inaccuracies and inefficiencies in analysis.
- Need for Skilled Personnel: Effective data utilization requires skilled personnel who can collect, analyze, and interpret data to support decision-making. This involves expertise in data analysis, statistical modeling, data visualization, and data management. Without trained personnel, data can become a costly resource that isn’t used to its full potential. Consider the cost of hiring or training data analysts, and the return on investment in their expertise.
Data Breaches and Misuse Mitigation
Protecting sensitive data is paramount. Agents must implement robust security measures to prevent data breaches and misuse. A proactive approach to risk mitigation is essential.
Potential Risks | Mitigation Strategies |
---|---|
Unauthorized Access | Implementing strong passwords, multi-factor authentication, and regular security audits. Employing encryption techniques for data transmission and storage. |
Data Loss | Regular data backups and disaster recovery plans. Utilizing cloud storage solutions with robust redundancy features. |
Data Manipulation | Establishing clear data access controls and user permissions. Implementing audit trails to track data modifications. |
Malicious Use | Educating staff on data security best practices and potential threats. Implementing intrusion detection systems to identify and respond to malicious activities. |
Data breaches can have severe consequences, including financial losses, reputational damage, and legal repercussions. Thorough risk assessments and the implementation of robust security measures are crucial to mitigating these risks.
Case Studies
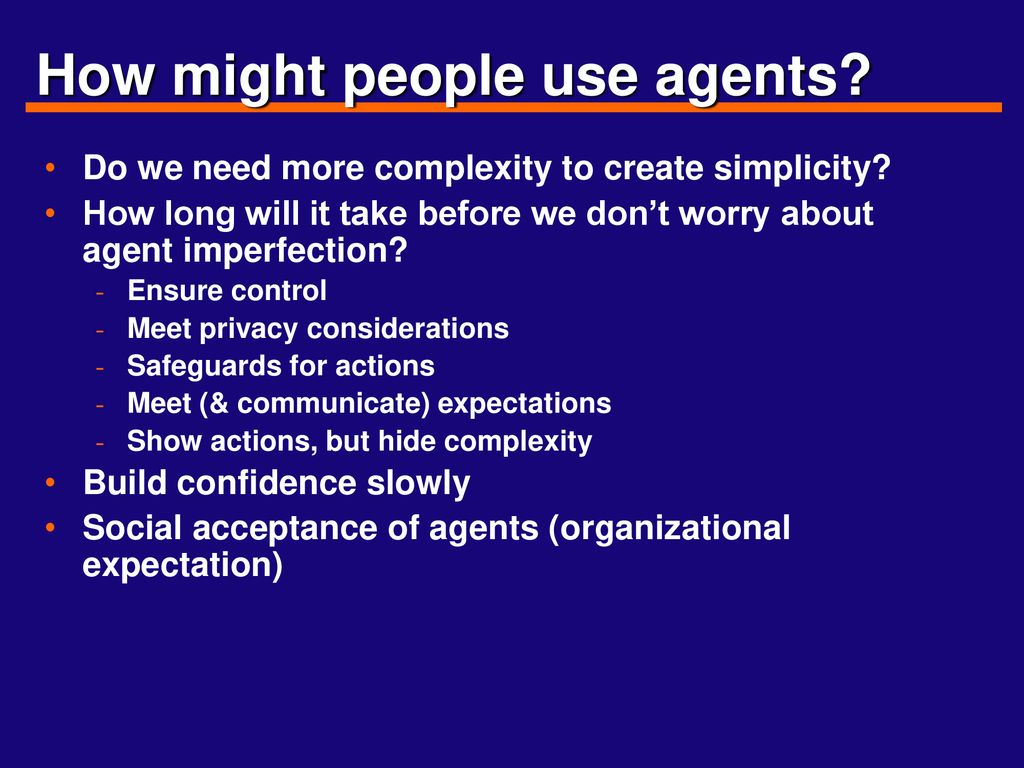
Data-driven decision making isn’t just a buzzword; it’s a powerful tool for agents across various industries. Real-world examples showcase how harnessing data can lead to significant improvements in performance, efficiency, and customer satisfaction. These case studies provide valuable insights into the practical application of data analysis and the tangible results achieved.Effective data utilization goes beyond collecting information; it’s about understanding the stories within the data and translating them into actionable strategies.
Travel agents need to really embrace data analysis. Knowing what’s popular, like the enhanced activities on the Avalon ship, activities amped up on avalon ship , is key. This helps tailor perfect trips for clients, and ultimately, drives better bookings. It’s all about using data to predict and respond to client needs. This data-driven approach is vital for success in the travel industry.
The following examples demonstrate how agents have transformed their operations by embracing a data-driven approach.
Real-World Examples of Data-Driven Success
Data-driven strategies are not theoretical concepts; they translate into measurable improvements in agent performance. These case studies demonstrate the impact of data-driven approaches on various aspects of agent operations.
Agent | Data Used | Results Achieved | Lessons Learned |
---|---|---|---|
Customer Service Team at a Retail Bank | Customer interaction logs, transaction history, and feedback surveys | Reduced customer complaints by 15%, improved first-call resolution rate by 10%, and increased customer satisfaction scores by 8%. | Analyzing customer interaction data helped identify recurring issues and train agents to address them effectively. Using feedback surveys allowed the bank to proactively address areas for improvement in their service. |
Sales Team at a Software Company | Lead qualification data, sales conversion rates, and customer demographics | Increased sales conversion rate by 20% and reduced sales cycle time by 15%. | Identifying high-value leads and tailoring sales pitches based on customer data significantly improved the efficiency and effectiveness of the sales process. |
Technical Support Team at an E-commerce Platform | Customer support ticket data, product usage data, and error logs | Reduced average resolution time for technical support issues by 25% and improved customer satisfaction scores by 10%. | Analyzing data helped identify common technical issues, allowing for the development of more comprehensive and efficient solutions to improve the support process. Prioritization of recurring issues allowed for targeted solutions and training. |
Key Patterns and Best Practices
These case studies reveal several key patterns and best practices that agents can adopt to maximize the impact of data-driven strategies.
- Data Collection and Integration: Effective data collection and integration across various sources are crucial. This requires careful planning and consistent data management practices.
- Data Analysis and Interpretation: Sophisticated data analysis tools and skilled personnel are essential to derive actionable insights from data. Interpretation should focus on identifying trends and patterns to inform decision-making.
- Data-Driven Action and Improvement: Data-driven strategies must lead to tangible actions. This includes process improvements, training programs, and adjustments to workflows. These changes should be tracked to ensure effectiveness.
- Continuous Monitoring and Evaluation: Regular monitoring and evaluation of the impact of data-driven strategies are essential to ensure ongoing improvements. This allows for adjustments to strategies based on performance.
Future Trends
The future of data-driven decision making for agents is brimming with exciting possibilities. As data volumes continue to explode and new technologies emerge, agents who embrace these trends will gain a significant competitive edge. This section explores emerging trends in data analysis, highlighting potential impacts on agents and innovative applications.
Emerging Trends in Data Analysis
The field of data analysis is rapidly evolving, with new techniques and technologies constantly emerging. Machine learning algorithms are becoming increasingly sophisticated, enabling agents to uncover hidden patterns and insights from complex datasets. This advancement allows for more accurate predictions and proactive decision-making. Natural Language Processing (NLP) is also playing a crucial role, enabling agents to interpret and extract meaning from unstructured data like customer reviews or social media posts.
This deeper understanding of customer sentiment and market trends allows agents to adapt their strategies in real-time.
Emerging Technologies Enhancing Data-Driven Approaches
Several emerging technologies are poised to revolutionize data-driven approaches for agents. The rise of cloud computing allows for scalable and cost-effective data storage and processing. This enables agents to handle massive datasets and perform complex analyses without significant infrastructure investments. Edge computing, processing data closer to its source, enables faster response times and reduced latency in data-driven applications.
This is especially crucial for real-time decision-making in areas like fraud detection and customer support. Furthermore, the increasing availability of open-source data and tools empowers agents with greater accessibility to advanced data analysis techniques.
Future of Data Management and Agent Empowerment
Data management is critical to realizing the full potential of data-driven approaches. The future of data management emphasizes data quality, security, and accessibility. Robust data governance frameworks are essential to ensure compliance with regulations and maintain trust. Advanced data visualization tools will allow agents to interpret complex data insights in a clear and understandable manner. Data lakes and data warehouses will store and manage heterogeneous data sources, enabling more comprehensive analyses and predictive modeling.
This will provide agents with the information they need to make better decisions, increasing their efficiency and effectiveness.
Innovative Applications of Data Analysis for Agents
The future will see numerous innovative applications of data analysis in agent roles. For example, in customer service, predictive analytics can be used to anticipate customer needs and proactively resolve issues before they escalate. In marketing, data-driven segmentation can enable targeted campaigns that yield higher conversion rates. In fraud detection, real-time anomaly detection can help identify fraudulent transactions and prevent losses.
Personalized recommendations based on individual customer preferences are another potential application, enhancing customer satisfaction and loyalty.
Future Trends in a Table
Trend | Potential Application | Implications for Agents |
---|---|---|
Advanced Machine Learning | Predictive modeling for customer churn, fraud detection, and personalized recommendations. | Improved efficiency, reduced costs, and enhanced decision-making. |
Cloud Computing | Scalable data storage and processing for large datasets. | Reduced infrastructure costs and increased access to advanced tools. |
Edge Computing | Real-time data processing and analysis for applications like fraud detection and customer support. | Faster response times and more effective real-time decision-making. |
Data Visualization | Clear and understandable representation of complex data insights for better understanding. | Enhanced comprehension of data and improved communication with stakeholders. |
Open-Source Data & Tools | Increased accessibility to advanced data analysis techniques. | Lower barriers to entry for adopting data-driven approaches. |
Outcome Summary
In conclusion, the power of data is undeniable for agents seeking to succeed. By understanding the value of data collection, analysis, and interpretation, agents can make informed decisions, optimize performance, and adapt to changing market landscapes. The future of data-driven approaches is bright, and agents who embrace these strategies will undoubtedly achieve significant improvements. Remember, the key is not just to collect data, but to effectively use it to gain actionable insights and drive positive change.
Question & Answer Hub: Agents Must Harness The Power Of Data
What are some common data sources agents can utilize?
Agents can leverage a variety of data sources, including market research reports, customer relationship management (CRM) systems, sales figures, website analytics, and social media trends. Each source provides unique insights that can be combined to paint a comprehensive picture.
What are the potential risks of using data improperly?
Data breaches, inaccurate information, and misuse of data are significant risks. Understanding these potential pitfalls and implementing robust security measures are crucial for responsible data handling.
How can agents ensure data quality and accuracy?
Data quality and accuracy are paramount. Employing data validation techniques, verifying data sources, and establishing clear data governance procedures can ensure reliability and minimize errors.