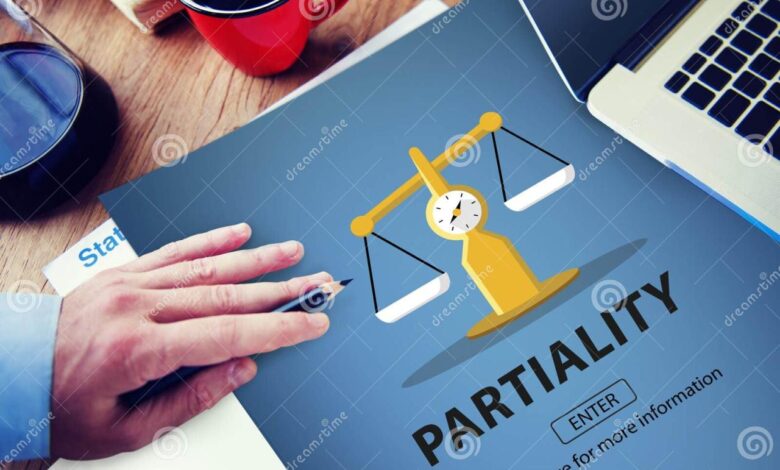
Understanding Bias Types and Impacts
Bias sets the stage for many of the challenges we face in today’s world, from skewed decision-making to misrepresented data. This exploration delves into the various forms of bias, examining how they manifest in different contexts and their far-reaching consequences.
We’ll explore the different types of bias, from the subtle cognitive biases that influence our everyday choices to the systemic biases embedded in institutions and societal structures. This includes examining the impact of bias on everything from data analysis to media representation and even algorithmic decision-making. Understanding bias is crucial to building a more equitable and just world.
Defining Bias
Bias, in its broadest sense, is a systematic predisposition to perceive, interpret, and act in ways that favor certain outcomes or perspectives over others. It’s a fundamental aspect of human cognition, often influencing our judgments and decisions without our conscious awareness. Understanding bias is crucial for recognizing its potential impact on individual interactions and societal structures.Cognitive biases are tendencies to make systematic errors in judgment and reasoning.
We all have biases, whether conscious or not. It’s fascinating to consider how these ingrained preferences might influence someone like a top-tier executive chef, like the one profiled in a day in the life hal executive chef. From sourcing ingredients to managing staff, their daily choices could reflect their personal biases, which might in turn affect the overall experience for their team and customers.
Ultimately, understanding these biases is key to promoting a more inclusive and equitable environment.
These errors are often caused by shortcuts or heuristics the mind employs to simplify complex information processing. Confirmation bias, for instance, is a common cognitive bias where individuals tend to seek out and favor information that confirms their existing beliefs, while ignoring or downplaying contradictory evidence.
Types of Bias
Various types of bias exist, each with its own characteristics and potential consequences. Understanding these diverse forms is essential for mitigating their impact.
- Cognitive Bias: These biases stem from the way our minds process information. They represent systematic patterns of deviation from norm or rationality in judgment. Examples include anchoring bias (over-reliance on initial information), availability bias (overestimating the likelihood of events that are easily recalled), and framing bias (different responses to the same information depending on how it’s presented).
- Confirmation Bias: This bias involves actively seeking and interpreting information that confirms pre-existing beliefs. A person might only read news articles that align with their political views, thus reinforcing their existing perspectives. This bias is pervasive and can lead to the reinforcement of inaccurate or incomplete beliefs.
- Implicit Bias: This refers to unconscious attitudes and stereotypes that affect our understanding, actions, and decisions. These biases are often rooted in societal norms and experiences, and can lead to discriminatory behavior even if the individual consciously rejects prejudice. For instance, a seemingly neutral hiring manager might unconsciously favor candidates with names associated with certain demographics. The impact of implicit bias is substantial, potentially leading to disparities in opportunity and outcomes.
- Anchoring Bias: This bias occurs when individuals rely too heavily on the first piece of information they receive (the “anchor”) when making decisions. For example, a car’s initial listing price can heavily influence a buyer’s perception of its fair market value, even if subsequent information suggests a different price.
Intentional vs. Unintentional Bias
It’s crucial to distinguish between intentional and unintentional bias. Intentional bias involves deliberate and conscious prejudice. Unintentional bias, on the other hand, arises from ingrained stereotypes, heuristics, and cognitive shortcuts, without conscious intent to discriminate. Both types of bias can have detrimental effects, although unintentional bias may be more subtle and pervasive in its influence.
Impact of Implicit Bias
Implicit bias can have a profound impact on various aspects of life. It can lead to disparities in areas such as employment, housing, education, and the criminal justice system. For example, research has shown that implicit racial bias can influence how judges sentence defendants.
Common Biases and Consequences
Bias Type | Description | Example | Impact |
---|---|---|---|
Confirmation Bias | Seeking out information that confirms existing beliefs. | Ignoring data contradicting a theory. A scientist only analyzing data that supports their hypothesis, neglecting conflicting results. | Reinforcement of inaccurate beliefs and hindered progress in research or decision-making. |
Availability Heuristic | Overestimating the likelihood of events that are easily recalled. | Someone believing plane crashes are more common than car accidents due to vivid media coverage of plane crashes. | Incorrect risk assessments and potentially irrational decisions. |
Implicit Bias | Unconscious attitudes and stereotypes affecting judgments and actions. | A manager unconsciously favoring candidates from a similar background to their own. | Disparities in opportunities and outcomes based on social group membership. |
Sources of Bias
Information systems, while powerful tools, can perpetuate existing societal biases. These biases, stemming from various sources, can subtly influence outcomes and create unfair or discriminatory results. Understanding these sources is crucial for building fairer and more equitable systems.The presence of bias in information systems isn’t simply a technical issue; it reflects broader societal inequalities. Recognizing these underlying biases and actively working to mitigate them is a critical step towards building more just and representative technologies.
Societal Factors Contributing to Bias
Societal norms and values often shape the data used to train algorithms and the design of information systems. These norms, which can include stereotypes and prejudices, are ingrained in our cultures and frequently go unnoticed. These biases are not always malicious, but they can still lead to significant inequities. For instance, a system trained on historical data might reflect existing societal inequalities, leading to biased outcomes.
Individual Bias in Information Systems
Individuals involved in the design, development, and use of information systems can introduce their own biases. This includes developers who might subconsciously favor certain groups or make assumptions that reflect their own experiences. The choice of data used to train algorithms and the design of user interfaces can also reflect personal biases. For example, a developer might design a facial recognition system that is less accurate for individuals with certain ethnicities or gender expressions.
Speaking of bias, it’s interesting how quickly things can shift when unexpected events occur. For example, airline and cruise line adjustments to their schedules due to Sandy’s impact highlight how quickly priorities can change and how that can influence decisions. airlines cruise lines alter plans due to sandy demonstrates how external factors can trigger significant changes, potentially leading to further biases in the way resources are allocated and how people react.
Ultimately, we can see bias at play in how we perceive and respond to such disruptions.
Systemic Bias in Information Systems
Systemic bias arises from flaws in the design and implementation of information systems themselves. These systems can inadvertently perpetuate societal biases through their inherent structure, data sets, or algorithms. Examples include biased datasets that overrepresent certain groups or underrepresent others, leading to inaccurate or unfair outcomes. Algorithms trained on biased data can also perpetuate and even amplify these biases, further widening existing inequalities.
The Role of Cultural Norms in Perpetuating Bias
Cultural norms play a crucial role in shaping perceptions and expectations, which in turn influence the design and use of information systems. Stereotypes and prejudices often embedded within cultural norms can lead to biased algorithms and outcomes. For instance, if a system is trained on data that reflects historical gender roles, it might perpetuate biases against women in the workplace or other areas.
Table Demonstrating Sources and Types of Bias
Source | Type of Bias | Example |
---|---|---|
Cultural Norms | Gender Bias | Algorithms trained on historical data reflecting traditional gender roles may underrepresent women in leadership positions. |
Individual Bias | Racial Bias | A developer might subconsciously favor certain ethnicities in the design of an algorithm for loan applications, leading to discriminatory outcomes. |
Systemic Bias | Algorithmic Bias | A facial recognition system trained on a dataset that predominantly includes images of light-skinned individuals might perform less accurately on individuals with darker skin tones. |
Societal Factors | Class Bias | A system used to predict future criminal behavior trained on data that reflects historical inequalities in policing might disproportionately label individuals from marginalized communities as high-risk. |
Bias in Different Contexts
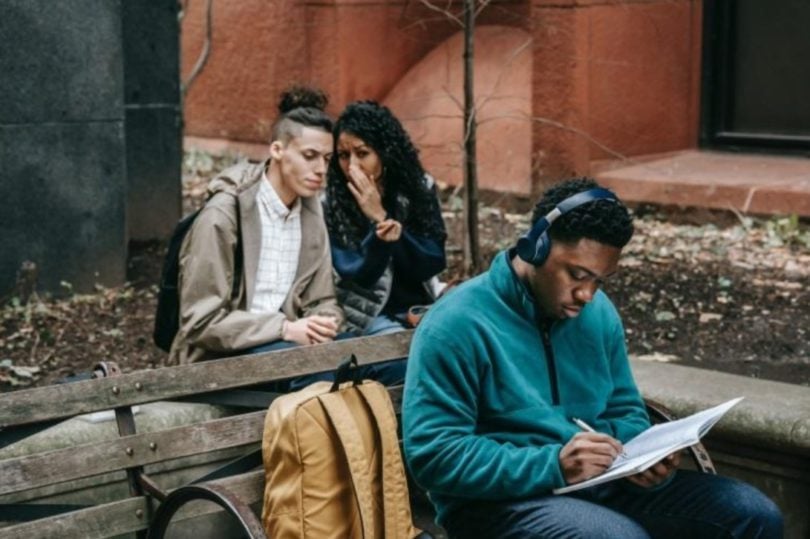
Bias isn’t confined to a single domain; it permeates various aspects of human interaction and decision-making. From the subtle prejudices embedded in our data collection methods to the overt narratives presented in media, bias manifests in countless ways. Understanding these diverse forms of bias is crucial for fostering objectivity and promoting fairness in all spheres of life.Bias significantly impacts how we perceive information, make choices, and interact with the world around us.
Recognizing the existence and manifestations of bias is a vital step towards mitigating its detrimental effects and striving for a more equitable and just society.
Bias in Decision-Making Processes
Decision-making processes, whether personal or institutional, are often susceptible to various forms of bias. Cognitive biases, like confirmation bias, can lead individuals to favor information that confirms their existing beliefs while overlooking contradictory evidence. Anchoring bias influences decisions based on the initial information received, even if that information is irrelevant or unreliable. These biases can significantly skew judgment and lead to suboptimal outcomes.
For example, a hiring manager might favor candidates who resemble their own background, overlooking equally qualified candidates from diverse backgrounds.
Bias in Data Collection and Interpretation
Data collection and interpretation are critical components of numerous fields, from scientific research to market analysis. Bias can creep into these processes at several stages, affecting the reliability and validity of the resulting conclusions. Sampling bias occurs when the sample used to represent a population isn’t truly representative, leading to inaccurate generalizations. Confirmation bias can also influence the selection and interpretation of data, leading to skewed results.
For example, in a study on the effectiveness of a new drug, researchers might unconsciously focus on data supporting the drug’s effectiveness while ignoring or downplaying contradictory findings.
Bias in Media Representation
Media plays a significant role in shaping public perception and understanding of the world. Representation bias in media can result in a skewed and incomplete picture of reality. Underrepresentation of specific groups, either based on ethnicity, gender, or socioeconomic status, can perpetuate stereotypes and limit public understanding of diverse perspectives. This can lead to misinformation, prejudice, and social inequalities.
It’s easy to fall into biases when judging things, isn’t it? Especially when it comes to events like Asta in New York asta in new york. The sheer scale and hype surrounding the event can sometimes overshadow the smaller details, creating a pre-conceived notion. Ultimately, a bit of critical thinking helps us avoid those biases and appreciate the unique aspects of any event.
For instance, news coverage disproportionately focusing on certain political viewpoints can create a biased understanding of the political landscape for viewers.
Bias in Algorithms and AI Systems
Algorithms and AI systems are increasingly used in various applications, from loan approvals to criminal justice decisions. Bias in these systems can arise from the data they are trained on. If the training data reflects existing societal biases, the algorithm will likely perpetuate and amplify those biases in its output. For example, facial recognition software might perform less accurately on individuals with darker skin tones due to bias in the training dataset.
This can lead to inaccurate identification and potentially discriminatory outcomes.
Table Contrasting Bias in Different Contexts
Context | Type of Bias | Example | Impact |
---|---|---|---|
Decision-Making | Confirmation Bias | Hiring managers favoring candidates who share their background. | Potential for overlooking qualified candidates from diverse backgrounds. |
Data Collection | Sampling Bias | A survey on public opinion only surveying residents of one city. | Inaccurate representation of public opinion across the entire region. |
Media | Representation Bias | News coverage disproportionately focusing on a specific political party. | Skewed perception of the political landscape. |
Algorithms/AI | Algorithmic Bias | Facial recognition software performing less accurately on individuals with darker skin tones. | Potential for discriminatory outcomes in applications like security or law enforcement. |
Addressing Bias
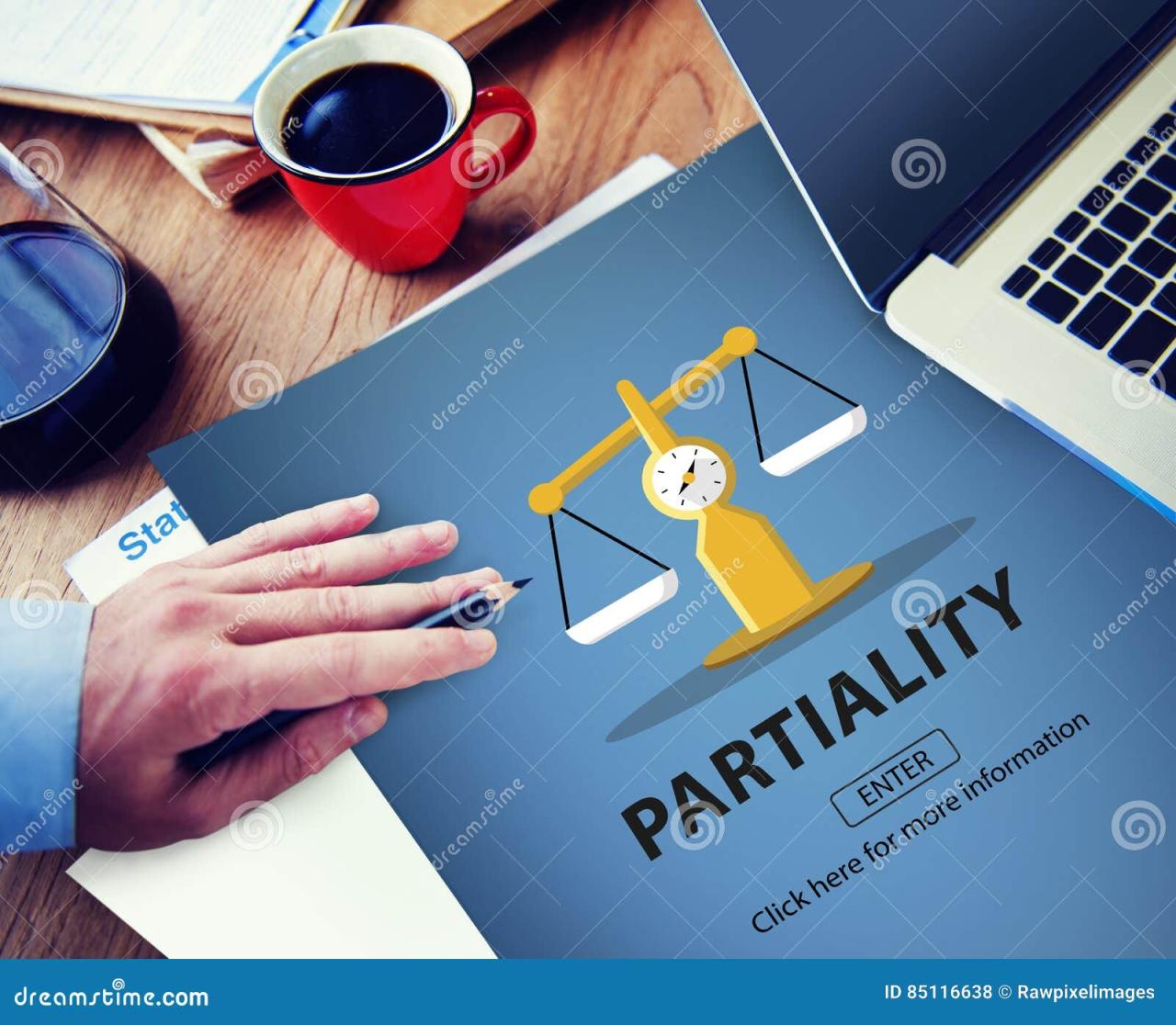
Uncovering and mitigating bias is crucial for creating fair and equitable systems in all aspects of life, from data analysis to algorithmic design. Addressing bias requires a multifaceted approach that combines awareness, education, and concrete strategies for identifying and eliminating prejudiced outcomes. Understanding the various forms of bias and their potential impacts is the first step towards creating a more just and inclusive world.Bias isn’t simply a problem in academic or scientific research; it pervades our daily interactions and influences decisions in everything from hiring practices to loan applications.
By proactively addressing bias in its many forms, we can foster environments that are more representative, equitable, and conducive to growth and progress for everyone.
Identifying and Mitigating Bias in Various Fields
Identifying bias in different fields requires careful consideration of the specific context. In the legal system, for example, implicit biases can influence judges’ decisions, potentially leading to unfair outcomes. Similarly, in the healthcare sector, unconscious biases can affect the quality of care received by certain patient groups. Careful analysis of existing data and protocols, along with training programs, can help identify and mitigate such biases.
For instance, using blind reviews in academic publishing or employing diverse panels in hiring processes are examples of strategies for reducing bias in specific fields.
Reducing Bias in Data Analysis
Data analysis is a powerful tool, but it can also perpetuate existing biases if not handled carefully. Biased data sets can lead to skewed results and perpetuate unfair or inaccurate conclusions. Data scientists need to be vigilant about the potential for bias in the data they use and employ techniques like data validation and diversity in data representation to address this issue.
- Careful data collection: Ensure data collection methods are free of bias. For instance, using stratified sampling can help to ensure that different groups in the population are represented in the dataset. Sampling techniques should consider factors that could influence the data, such as demographics, socioeconomic status, or geographical location.
- Data validation: Scrutinize the data for inconsistencies or patterns that might indicate bias. Outliers or unusual data points should be investigated to understand their source and impact on the analysis.
- Data standardization: Use standardized techniques to ensure that different data points are measured and compared on an equal footing. This can help to minimize the impact of variations in measurement scales or units.
Designing Unbiased Algorithms
Algorithms are increasingly used in decision-making processes, and if not designed carefully, they can perpetuate existing biases. Designing unbiased algorithms requires careful consideration of the data used to train the algorithm, as well as the potential impact of the algorithm’s output.
- Fairness-aware algorithms: Researchers are developing algorithms specifically designed to mitigate bias. These algorithms take into account the potential for bias and adjust their output accordingly. For instance, algorithms designed for loan applications could be adjusted to account for factors that might historically discriminate against certain groups.
- Transparency and explainability: It’s important that algorithms are transparent and explainable. This allows for scrutiny and identification of potential biases within the algorithm’s decision-making process.
- Regular monitoring and evaluation: Algorithms should be regularly monitored and evaluated for bias. This involves tracking the algorithm’s performance across different groups and identifying any potential disparities.
The Role of Education and Awareness
Education and awareness are fundamental to overcoming bias. Promoting critical thinking skills and providing bias awareness training can equip individuals with the tools and understanding needed to identify and mitigate bias in their own actions and decisions.
- Promoting diverse representation: Diversity in workplaces, educational institutions, and other settings can help to challenge existing biases and promote a more inclusive environment.
- Encouraging critical thinking: Critical thinking skills help individuals to evaluate information objectively and to recognize potential biases in themselves and others.
- Providing bias awareness training: Training programs can raise awareness about the existence and impact of biases, providing individuals with strategies to mitigate them in their professional and personal lives.
Steps to Create an Inclusive Environment
Creating an inclusive environment free from bias requires a concerted effort from individuals and organizations. A multi-pronged approach encompassing diverse representation, critical thinking, and bias awareness training is crucial.
- Promote diverse representation: Actively recruit and retain individuals from diverse backgrounds to ensure representation at all levels of an organization or institution.
- Encourage critical thinking: Foster an environment where individuals feel empowered to question assumptions and challenge existing norms.
- Provide bias awareness training: Offer regular training to raise awareness of biases and provide strategies for mitigating their impact in various contexts.
Bias in Data
Data, the lifeblood of modern decision-making, is often susceptible to biases. These biases, reflecting the inherent biases of the data collectors, processors, and users, can lead to skewed outcomes and perpetuate unfair or inaccurate representations of reality. Understanding how bias enters datasets, how to detect it, and how to mitigate its effects is crucial for building trustworthy and equitable systems.The presence of bias in data can have far-reaching consequences.
In machine learning, biased datasets can lead to discriminatory outcomes, such as biased loan applications or inaccurate medical diagnoses. In market research, skewed data can result in inaccurate projections, affecting investment decisions and strategic planning. Recognizing and rectifying these biases is paramount for building fairer and more representative models.
How Bias is Introduced into Datasets
Bias can be introduced into datasets at various stages, from data collection to data analysis. Inadequate sampling methods can result in underrepresentation of specific groups, leading to a skewed perspective. For instance, if a survey about consumer preferences is conducted primarily in urban areas, it may fail to capture the diverse needs and preferences of rural populations. Furthermore, inherent biases in the questions themselves can influence responses, creating a biased dataset.
Data collected from individuals with pre-existing biases might reflect those biases, and the data collection process might inadvertently reinforce those biases.
Methods for Detecting Bias in Data
Detecting bias in data requires a systematic approach. Data auditing, a thorough review of the dataset for disparities, is a vital first step. This involves scrutinizing the data for imbalances in representation across various demographic or categorical variables. For example, if a dataset about job applications reveals a significant disparity in the acceptance rate for men versus women, it indicates a potential bias.
Statistical analysis can also uncover patterns that suggest bias. Statistical tools can highlight anomalies or unexpected trends that point towards underrepresentation or overrepresentation of certain groups.
Techniques for Mitigating Bias in Datasets
Mitigating bias in datasets requires proactive measures at various stages. Ensuring representative sampling is crucial. Researchers should strive to include participants from diverse backgrounds and demographics to avoid underrepresentation. Data cleaning and preprocessing techniques can help address issues arising from biased data collection methods. This includes identifying and correcting inconsistencies or errors that might reflect or reinforce biases.
Using diverse and representative datasets is paramount. Including individuals from various backgrounds, experiences, and viewpoints can enhance the validity and reliability of the dataset, thus reducing the likelihood of biased outcomes.
The Importance of Diverse and Representative Datasets
Diverse and representative datasets are essential for building accurate and equitable models. By incorporating a wider range of perspectives, these datasets can better reflect the complexity of the real world. This leads to more accurate and reliable predictions and outcomes. For example, a dataset about customer preferences that includes a broad spectrum of ages, ethnicities, and socioeconomic backgrounds is more likely to yield accurate predictions about market trends and consumer behavior.
Comparing Methods for Detecting and Mitigating Bias
Method | Description | Example |
---|---|---|
Data Auditing | Systematic review of data for biases, involving a thorough examination of the dataset for imbalances in representation across demographic or categorical variables. | Checking for gender or racial disparities in a dataset of loan applications. |
Statistical Analysis | Employing statistical methods to identify patterns that suggest bias, such as anomalies or unexpected trends in data distributions. | Using hypothesis testing to determine if there’s a significant difference in success rates between different demographic groups in a job application dataset. |
Data Cleaning and Preprocessing | Identifying and correcting inconsistencies or errors in data that might reflect or reinforce biases. | Removing irrelevant or inaccurate data points that might skew the results, such as duplicate entries or outdated information. |
Diverse and Representative Sampling | Actively recruiting participants from a variety of backgrounds and demographics to avoid underrepresentation of specific groups. | Conducting a survey with participants from diverse geographical locations, socioeconomic backgrounds, and cultural identities. |
Visual Representation of Bias
Bias, in its various forms, often operates subtly, making its impact difficult to perceive. Visual representations, however, can powerfully illustrate the consequences of bias, making them readily understandable and impactful. This section explores several visual tools for understanding bias in different contexts, specifically focusing on its impact on loan applications, data analysis, and broader societal consequences.
Impact of Bias on Loan Applications
A bar graph, with loan applications on the x-axis and approval rates on the y-axis, could clearly demonstrate bias. Two distinct groups, perhaps based on race or socioeconomic status, would be shown. The graph would reveal a significant difference in approval rates between the two groups, highlighting the discriminatory effect of bias. This visual representation would underscore how seemingly impartial processes can be profoundly influenced by hidden biases.
The graph would use color-coding to distinguish the groups and include labels for clear understanding.
We all have biases, don’t we? They can subtly influence our choices, even when we’re trying to be objective. For example, a desire for a truly relaxing, off-the-grid escape might lead you to seek out a place like the Aqua Nicaragua Eco Resort, offering a truly unplugged experience. Aqua Nicaragua Eco Resort offers unplugged escape But these biases, while often unconscious, can sometimes keep us from truly appreciating the diverse and amazing places in the world around us.
Bias Manifestation in a Dataset
A scatter plot, with loan application income on the x-axis and the likelihood of approval on the y-axis, could visually represent bias in a dataset. A cluster of points representing applicants with low incomes and low approval rates would stand out compared to a cluster of points with high incomes and high approval rates. This visual representation would show how certain demographics are disproportionately affected by the bias present in the dataset.
It’s easy to see bias in action, isn’t it? Sometimes, seemingly neutral things like transportation choices can be influenced by political agendas. Take Amtrak, for example, at the intersection of travel and politics – a fascinating study in how seemingly mundane things can be deeply politicized. amtrak at junction of travel and politics reveals how government funding and public perception can be shaped to serve specific political goals, highlighting the inherent bias in such situations.
Ultimately, it’s a reminder that even seemingly objective observations can be clouded by bias.
The plot should include a trend line to highlight the correlation between income and approval rates.
Cascading Effects of Bias
A flowchart illustrating the cascading effects of bias could start with an initial biased decision, such as a discriminatory loan application evaluation. The flowchart would then depict the subsequent effects, like limited access to capital, reduced economic opportunities, and the perpetuation of inequality across generations. Each step would be clearly labeled, connecting the initial bias to its long-term consequences.
The arrows would visually demonstrate the cause-and-effect relationships.
Detecting and Addressing Bias
A flow chart to detect and address bias in loan applications could begin with identifying potential bias indicators, like disparities in approval rates. Next, the flow chart would guide the user through steps like data analysis to pinpoint the sources of the bias, and then, implement strategies to mitigate the bias, such as auditing and training. The chart would include decision points and loops for continuous monitoring and adjustments.
The flow chart would be designed with clear and concise steps.
Impact of Bias in the Financial Sector (Infographic)
An infographic could visually depict the impact of bias in the financial sector by presenting a segmented pie chart. The segments would represent different demographics and their access to financial services. For example, a segment could represent individuals with limited access to loans due to historical bias, highlighting the disparities in the financial landscape. The infographic would also include supporting data, statistics, and relevant facts.
A timeline could show how bias has manifested and evolved within the sector over time, emphasizing the persistent nature of the problem.
Summary
In conclusion, bias is a multifaceted issue with far-reaching consequences. From its roots in individual cognition to its manifestation in societal structures, bias impacts everything from personal decisions to large-scale systems. By understanding the different types of bias, their sources, and their impact, we can work towards mitigating its effects and building a more just and equitable future.
Answers to Common Questions
What is the difference between explicit and implicit bias?
Explicit bias refers to conscious and openly held prejudices. Implicit bias, on the other hand, is unconscious and often unintentional. It operates outside of our awareness, yet it can significantly influence our actions and decisions.
How does bias affect data analysis?
Biased data can lead to inaccurate conclusions and flawed interpretations. If a dataset is not representative of the population it aims to describe, the results derived from it will likely be skewed and unreliable. Careful data collection and analysis techniques are necessary to minimize bias.
What are some practical steps to reduce bias in decision-making?
Adopting diverse perspectives, employing structured decision-making frameworks, and actively seeking out counterarguments are key steps in reducing bias in decision-making. Regular self-reflection and awareness training can also be valuable tools.
Can algorithms be unbiased?
While algorithms can be designed to minimize bias, it’s important to recognize that they can still reflect the biases present in the data they are trained on. Developing and implementing algorithms that are aware of potential biases and include diverse training data is crucial to mitigating this issue.